Data quality: the challenges and best practices of Data Quality Management
Data Quality is essential for companies, and is proving to be a major challenge for operational processes in areas such as marketing, finance and production. But what is Data Quality, and how can we guarantee it over the long term?
What is Data Quality?
Data Quality refers to the implementation of actions aimed at ensuring that the data in a company's information system is correct and reliable. Data is a company's most precious asset , guaranteeing the efficiency of its processes and the relevance of its decisions and actions. That's why it's so important to have full confidence in the quality of your data before using or distributing it.
The more data is "consumed", i.e. used by processes, the higher the quality of the data.
How do you measure data quality?
This quality is measured by "technical" and "business" rules. Technical rules represent criteria intrinsic to the data:
- Data completeness: is the data mandatory?
- Data validity: has the data been entered in the right format?
- Data freshness: when was the data entered?
Business rules complete the qualification of this data through a set of rules conditional on its typology and use. They define a functional value for the data, through consistency and accessibility criteria, for example.
This set of rules then defines the true quality of the data concerned.
The impact of poor data quality
Data quality issues are intrinsically linked to a company's business challenges. Indeed, data quality is a key issue for companies today, as it can present a major risk if neglected, impacting decision-making, operational and compliance processes, as well as the company's openness to digitalization.
The impact of poor data quality on decision-making processes
Most decisions taken in an organization are based on figures drawn up from a greater or lesser volume of data. If these data are not sufficiently qualitative, projects or investments can be launched on the basis of erroneous information. Conversely, a dashboard based on accurate and reliable information enables pertinent reflection and confident decision-making.
The impact of poor data quality on operational processes
Poor data quality is detrimental to business productivity and efficiency: incorrect invoicing due to a data entry error on a customer file, faulty traceability that makes it impossible to trace the origin of a manufacturing problem... This makes operations time-consuming, since there is a constant need to check the source of the problem and correct the information.
The impact of poor data quality on corporate compliance
In some sectors, regulations are becoming increasingly strict on the communication of certain information to customers, consumers and partners. Take, for example, the French Anti-Waste for a Circular Economy Act (AGEC): the law now governs the environmental claims made by brands, and makes it compulsory for consumers to be informed about certain characteristics of the products they buy. A company whose data is of poor quality may find it difficult to comply, either because of an error in the information communicated, or because of the difficulty of tracing this information in the information system.
The impact of poor data quality in the digital world
The advent of digital technology has forced companies to open up to the outside world and expose themselves, no longer containing their information solely to their internal organization: e-commerce, marketplace, corporate site... The use of poorly qualified data can then have harmful effects on a company's reputation.
Conversely, digital technology has also made it possible to obtain more frequent data on customers, such as web pages consulted or orders placed, enabling a better understanding of the customer, a more personalized experience with the brand, and thus an improved brand image.
Data Governance and Data Management for data quality
Data Governance and Data Management are closely related concepts, since they are each other's counterparts in the data lifecycle.
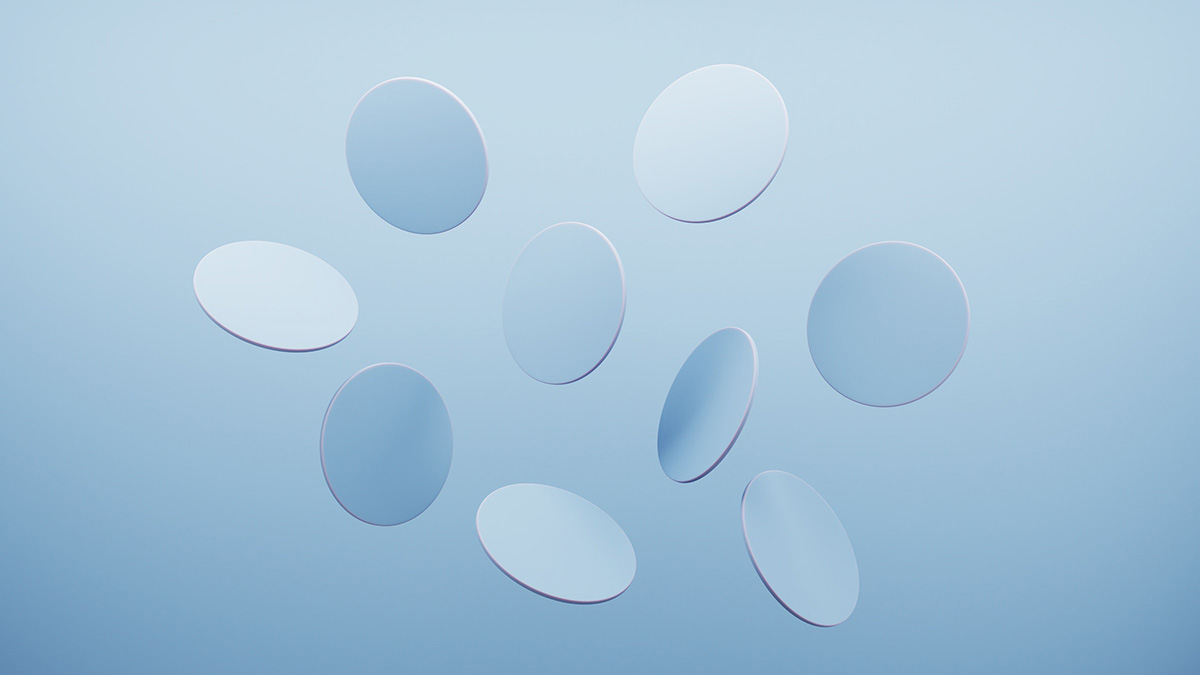
What is Data Governance?
Data Governance brings together all the practices and roles involved in obtaining, managing and using data. It confirms the quality and security of data throughout the company, and determines the roles and uses of this data. The decision to implement a Data Governance approach is most often taken after becoming aware of the impact of data quality.
The aim of data governance is not to change the way a company is organized, but to define a policy based on functional processes. That's why we define three governance models based on processes.
- Centralized governance model : used for product data, where information is top-down. The governance model is rather simple, but includes highly collaborative workflows involving many players and professions. Data processing is then centralized.
- Decentralized governance model : used in the context of customer data, where information flows upwards. This model is rather complex, since it concerns information that comes from the field, and therefore from several entry points. The aim of this governance model is to identify the "Golden Record", i.e. the version of a piece of data considered to be the "Point of Truth", in order to replenish systems with the right information. Data processing is then decentralized.
- Federated governance model : this governance model mixes centralized processing of high-value data with decentralized processing of low-value, high-volume data. The model redistributes roles, with a central team coordinating all departments, and each department managing its own governance.
What is Data Management?
Data Management refers to the technical and operational management of data. It covers the entire data lifecycle, including collection, validation, storage, protection, processing and enrichment. The aim is to ensure data reliability and accessibility.
There are three essential elements to Data Management:
- An adapted data architecture : this involves modeling the data lifecycle, in particular its collection and use. It is also in this architecture that data quality rules are integrated.
- A high-performance storage system : data must be recorded and stored so that it can be used. The aim of storing data, and above all storing it well, is to make it accessible to the right people in the company.
- Good data security : data security is essential, because as well as being a resource, it can also be a legal constraint. Data Management must therefore ensure that data is protected against cyber-attacks and complies with the legal requirements imposed by regulations.
Our Data Management partners
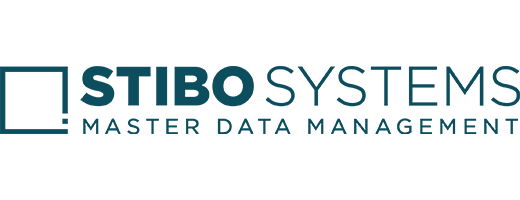
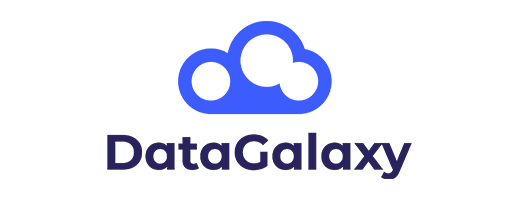
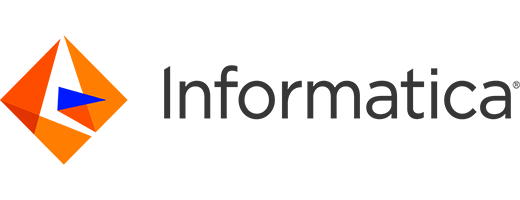
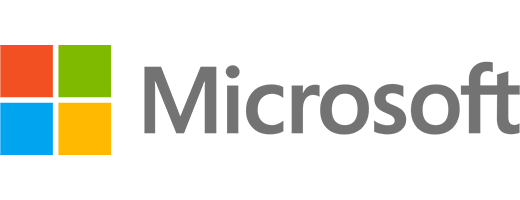
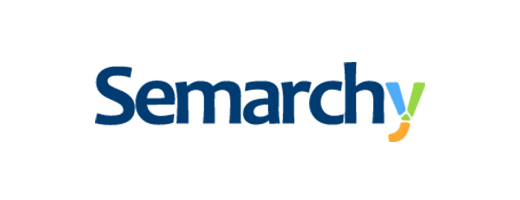
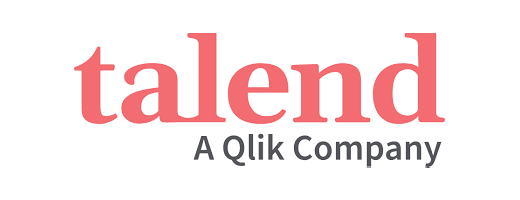
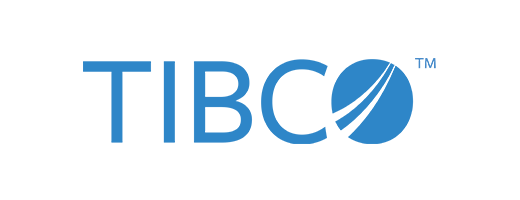
The relationship between AI and Data Quality
AI can do a lot. It can learn, listen, watch what we do with our data, and thus become an essential tool in improving data quality. Whether it's control, validation or enrichment, tasks that can be replicated, AI can accompany data players.
Bypassing deterministic rules
In the demanding field of Data Quality, where deterministic rules often dictate the standards, the introduction of AI represents a major evolution. Typically, quality control processes are limited by predefined rules. AI, on the other hand, offers a less deterministic approach, capable of learning new rules as it goes along. This adaptability can significantly improve data quality, particularly when processing large volumes of data. The real value lies in AI's ability to adjust dynamically and learn from past mistakes, offering an agile solution to validation and quality control challenges.
The challenges of data quality and control are considerable. Integrating AI is a strategic response to these challenges. By enabling AI to learn and apply new rules, companies can guarantee a superior level of quality, particularly in the context of processing large volumes of data. This approach not only promotes operational efficiency, but also a continuous improvement in data quality, reinforcing the reliability of information and facilitating more informed decision-making.
Data enrichment with AI
Data enrichment plays a crucial role in enhancing and complementing existing information, particularly important in the area of product data. Comprehensive data is not only essential for optimal product management, but also for an enriching customer experience. Qualifying thousands of products with attributes such as dimension, color and weight can become a tedious and error-prone task. Nevertheless, advances in generative AI offer a solution by making this process easier to implement, generating product descriptions or marketing pitches from a solid database.
The importance of good data quality to get started with AI
The connection between AI and data quality cuts both ways: AI can help maintain high data quality, while at the same time emphasizing that data quality is crucial for effective collaboration with AI. Whether for enrichment or information generation, it remains essential to recognize that AI requires a supply of information in order to learn and understand its tasks.
It's important to think of AI as a tool, an algorithm made available to professionals. Consequently, its effectiveness depends on it being fed correctly: if incorrect information is supplied to the AI, it is logical that it will generate elements of lower quality.
Data Quality players
Sensitivity to data quality within a company rests with those who use it on a daily basis. Marketing teams, responsible for campaigns; production, focused on manufacturing; digital, dedicated to the dissemination of information; and finance, focused on steering, are all key players responsible for the quality of the data they integrate into information systems.
For these business teams, data quality can be perceived as a subjective aspect that only becomes important when a specific process consumes it. It is only at this point that the question of data quality or non-quality becomes a reality, underlining the crucial importance of ensuring reliable and accurate data for efficient business operations.
Changing attitudes to data quality
There has been a significant shift in attitudes to data quality. When a company grows empirically, often through the association of several entities, each entity may have good quality data, but the lack of sharing and linking between repositories can lead to poor cross-functional quality, resulting in duplicate data.
As companies grow, the empirical approach, often based on tools such as Excel, reaches its limits. What was manageable at the outset quickly becomes unmanageable as the amount of data increases, underlining the need for a more systematic approach.
Also, compliance issues, such as the General Data Protection Regulation (GDPR), have prompted companies to question the documentation and measurement of data quality. It has become crucial to go beyond mere compliance and consider data as an essential value for overall business efficiency.
Change Management to support the implementation of Data Quality
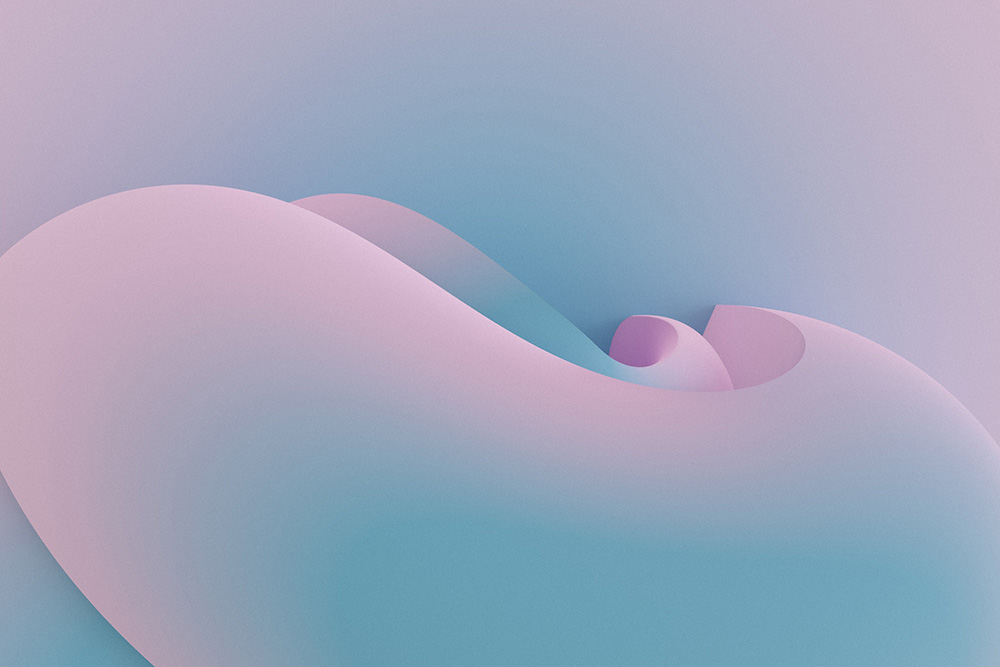
Change Management plays an essential role in supporting the implementation of Data Quality within a company. The success of any data quality initiative depends largely on how employees adopt and integrate these changes into their daily practices.
It is imperative to make teams aware of the benefits of Data Quality, highlighting how reliable data contributes to more efficient processes, informed decision-making and better overall company performance.
Employee buy-in is a central aspect of Change Management in the context of Data Quality. It involves making teams aware of the positive impact of data quality on their day-to-day activities, and actively involving them in the process. Listening to their concerns, creating an environment conducive to learning, and recognizing their efforts are key elements in creating a successful transition to a corporate culture where data quality is perceived as a strategic priority.
They trust us
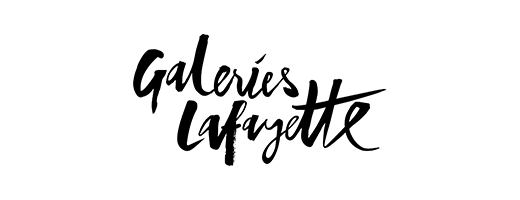
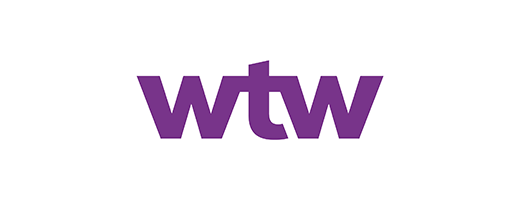
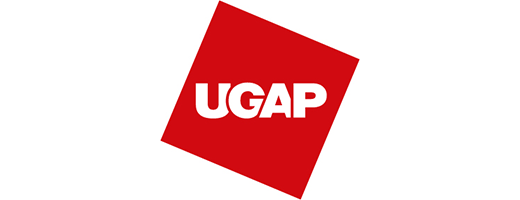
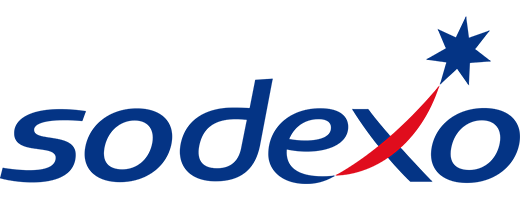
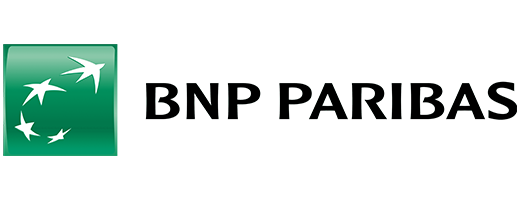
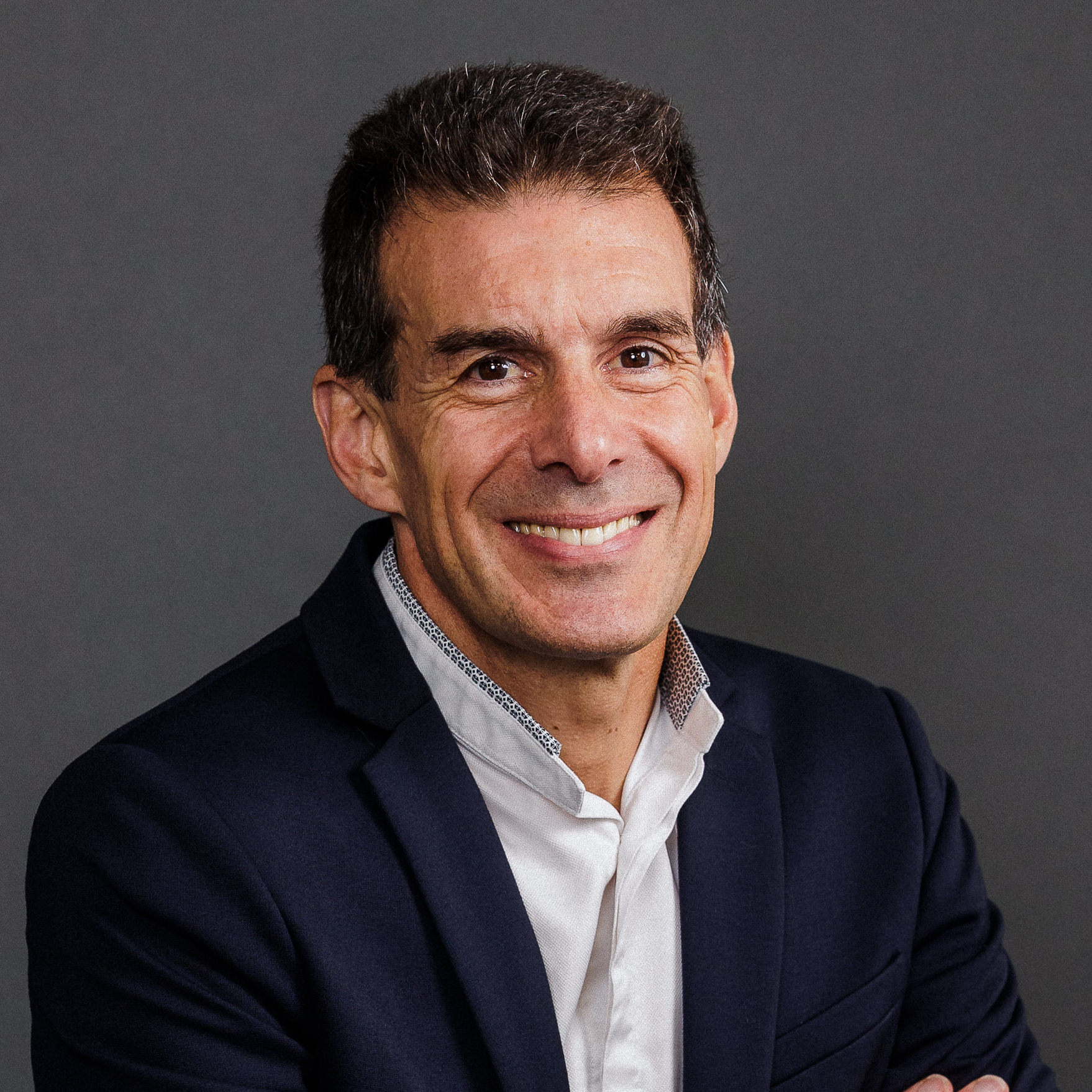
"Data quality is the fundamental foundation of business processes. In an ever-changing digital world, Data Governance and Data Management are the guardians of this quality, defining rules and processes that transcend organizational boundaries. By strategically integrating AI, we push the boundaries of traditional deterministic rules, offering unrivalled agility in the management and continuous improvement of data quality."
Micropole
In a nutshell
Data quality is essential to business success, impacting key sectors such as marketing, finance and production. Data Quality" aims to ensure the reliability of information system data, measured through technical and business rules. Poor data quality can have significant repercussions on decision-making, operational and compliance processes, as well as on a company's reputation and digital efficiency.
The integration of AI into data quality management represents a major breakthrough, enabling traditional deterministic rules to be bypassed. AI can enrich data, improving product management and customer experience. The relationship between AI and data quality is bidirectional, requiring the correct supply of quality data to ensure effective use of AI.
The key players responsible for data quality within a company are those who consume it, such as marketing, finance, production and digital teams. Changing mindsets around data quality has become crucial, particularly with increasingly stringent compliance requirements. Change Management plays an essential role in getting people on board and understanding the impact of the Data Quality approach.