Case study Micropole supports Crédit Agricole in fraud detection

×
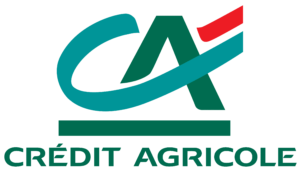
Micropole assists Crédit Agricole in the detection of bank card fraud
Find out how Micropole has enabled Crédit Agricole Payment Services (CAPS) to significantly optimize its real-time systems for fighting CB and flow fraud.
Context
Crédit Agricole is the largest network of cooperative and mutual banks in the world. In France, it is made up of 39 regional banks and has nearly 20 million customers with a bank card.
Each year within the group, several billion CB transactions are observed, for more than 150 million euros of attempted fraud.
Fraud is constantly evolving because fraudsters are constantly trying to outwit existing anti-fraud devices. It is therefore imperative to be constantly on the alert and to adapt your system on a daily basis.
Moreover, the operational constraints are strong since the system is based on rules that must decide in a few milliseconds whether or not to authorize a transaction.
Among the different types of fraud, Distance Selling is the most affected area.
Prior to Micropole's intervention, CAPS had tested several experiments to try to improve the existing system with new approaches based on machine learning and AI. These experiments, carried out with startups / editors / specialized firms, proved to be unsuccessful both in terms of their ability to stop new frauds and to integrate into the system under constraints
Challenges
Despite its previous unsuccessful experiments, Crédit Agricole Payment Services sought Micropole's support and expertise to demonstrate the significant contribution of Machine Learning and AI in determining new high-potential anti-fraud rules.
First, we focused on the scope of distance selling transactions (VAD). Indeed, these dematerialized and off-premises transactions are particularly prone to fraud and represent a significant part of the fraud suffered.
In summary, the mission initially consisted of demonstrating the ability of Machine Learning and AI to stop additional fraud in the mail order segment before generalizing the approach to all types of transactions / payments (withdrawals, proximity payments, transfers, checks).
Methods
Given the operational constraints, the rarity of fraud and the characteristics of current Machine Learning algorithms, Micropole's experts decided, after testing classic approaches, to develop a custom algorithm capable of automatically identifying new relevant rules against fraud (Scoring.AI and Fraud.AI).
This algorithm was designed to simulate, on past data, the ability of the rules identified over time (relearning the model at regular intervals) to stop fraud on future transactions.
Micropole quickly demonstrated the algorithm's ability to stop additional fraud on VAD transactions without generating additional inconvenience to customers.
In-depth work on the construction of new indicators with strong predictive potential for fraud has made it possible to increase initial performance in terms of fraud identified and therefore stopped.
After a first conclusive field trial lasting several months, CAPS asked Micropole to extend the approach to all transactions.
Benefits
- More than 10 million € of additional frauds stopped per year
- Device efficiency improved by 15%.
- No negative impact on the customer experience
- New algorithm specifically developed by Micropole, generating directly industrializable rules